A New Way to Think About AI Language Models
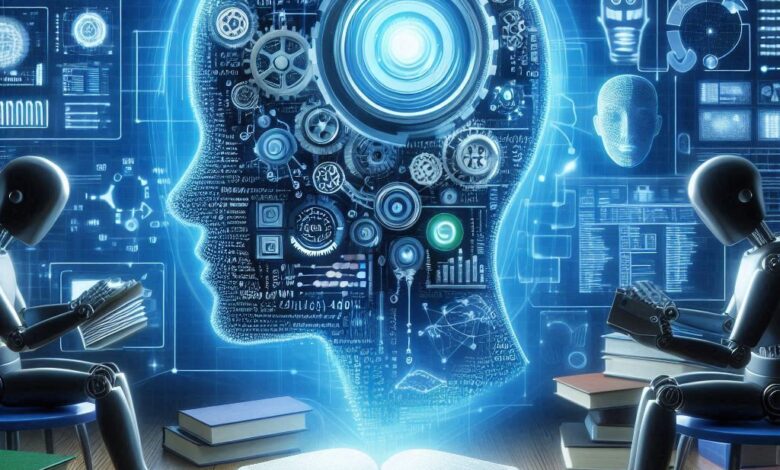
Artificial Intelligence (AI) has made huge strides in understanding and generating human language. You’ve probably heard of ChatGPT or other AI chatbots that can write essays, answer questions, and even crack jokes. But did you know these AI systems still have some big limitations? A researcher, Dr. Walid Saba published recently a paper in Academia titled “Towards Symbolic and Language-Agnostic Large Language Models” and he has some interesting ideas about how we might make them better. In this post, we will try to explain these ideas in a simplified way that is accessible for the general audience.
What’s the Problem with Current AI Language Models?
Current AI language models, despite being impressive, have some drawbacks:
- They’re Black Boxes: It’s hard to understand why these AIs say what they say. It’s like they’re thinking, but we can’t see their thought process.
- They Can Be Biased: Just like humans, these AIs can pick up and repeat unfair or hurtful ideas from the data they’re trained on.
- They Can Make Stuff Up: These AIs can sometimes confidently state things that aren’t true.
- They Miss Subtle Meanings: They sometimes misunderstand sentences where the exact meaning of words is crucial. For example, if you say “Mary wants to visit Paris,” the AI might think Mary wants to visit any big city in France, which isn’t necessarily true.
- They Struggle with Common Sense: Sometimes, these AIs miss the obvious interpretations that humans naturally understand. For instance, if you say “Every tourist visited two museums,” humans usually understand this to mean “many museums,” but an AI might not get this.
A New Approach: Teaching AI to Understand Language Like Humans Do
Dr. Saba suggests a new way of building AI language models. Instead of just feeding them lots of text, he proposes breaking down language into its basic building blocks. Here’s how it might work:
- Study How We Use Words: Look at how we use words in different situations to figure out what they really mean. For example, we might look at all the ways we talk about books: “The book changed my mind,” “The book is popular,” etc.
- Find Basic Relationships: Identify the fundamental ways we connect ideas in language. For instance, we often talk about things having properties (“The apple is red“) or being in certain states (“John is tired“).
- Build a Word Web: Create a network of how different words and ideas relate to each other. For example, we might notice that we can say a car or a computer is “running,” but we don’t say that about a couch. This tells us something about how these objects are different.
- Map Out Word Meanings: Describe the meaning of words by looking at all the ways we use them. For instance, we might describe a “book” as something that can change opinions, be popular, inspire movies, and so on.
- Consider How Common Different Uses Are: Note that some ways of using a word are more common than others. For example, we might say “an influential book” more often than “a heavy book,” so “influential” might be a more important part of what we mean by “book.”
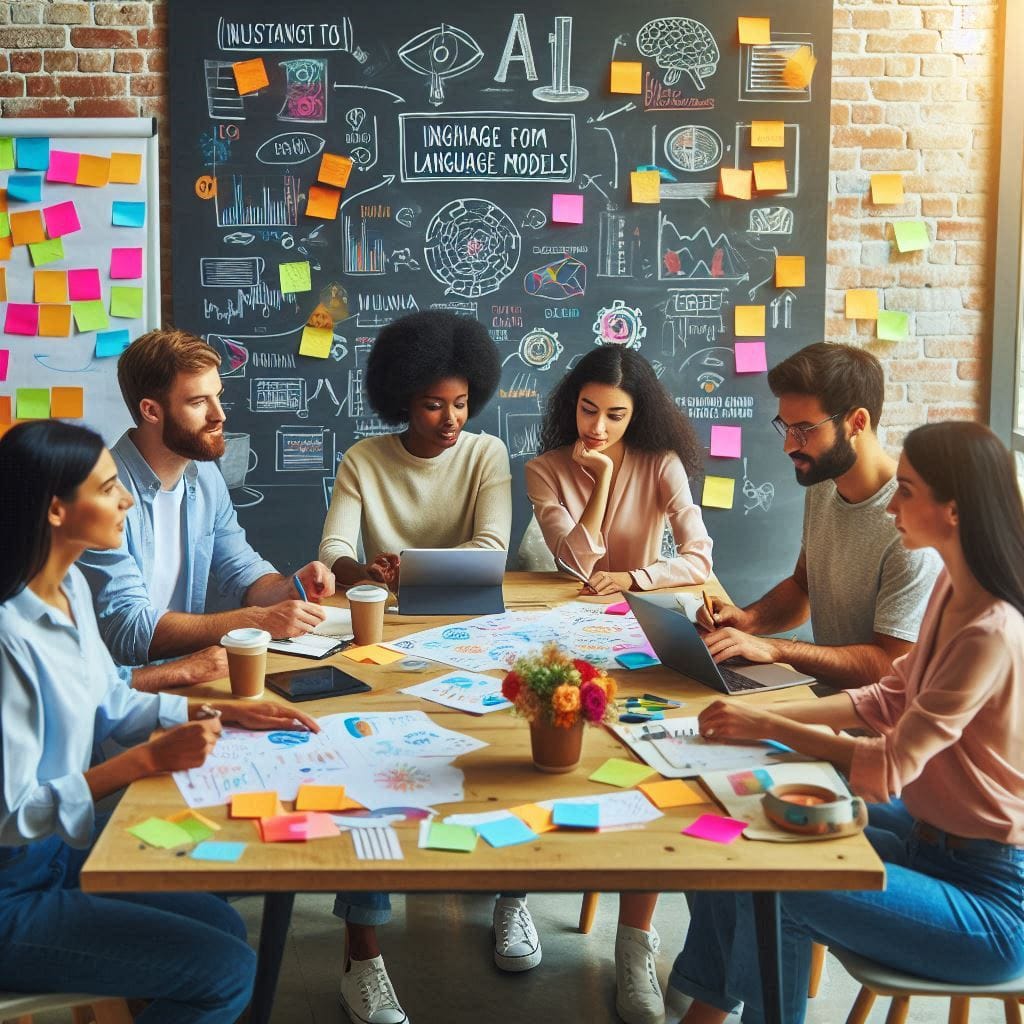
Why This New Approach Could Be Better?
This new way of building AI language models could have some big advantages:
- We Could Understand How It Works: Unlike current AI, we’d be able to see the reasoning behind its responses.
- It Could Work for Any Language: Because it focuses on basic ideas rather than specific words, it might work well across different languages.
- It Could Handle Tricky Language Better: It might be better at understanding things like metaphors and figures of speech.
- It Could Tell the Difference Between Ideas and Specific Things: For example, it would understand that while we can describe both books and magazines as “influential,” not every single book or magazine is actually influential.
While this new approach is still just an idea, it offers an exciting direction for making AI language models better. By combining what we’ve learned from current AI with this new way of breaking down language, we might be able to create AI that understands and uses language more like humans do.
As we keep pushing the boundaries of AI, it’s important to explore different approaches like the one suggested by Dr. Saba. They might help us overcome some of the big challenges we’re facing with current AI language models.
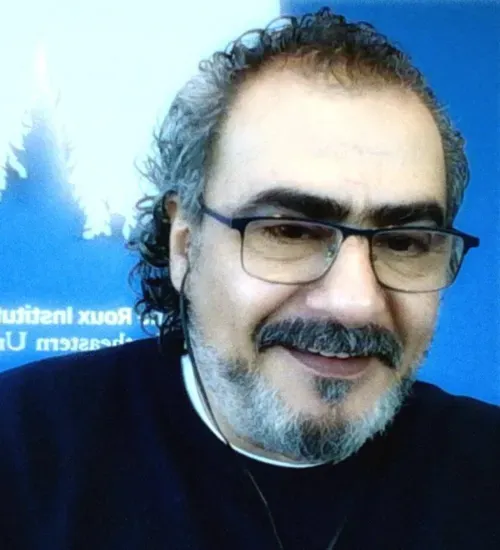
Who is Dr. Walid Saba?
Dr. Walid Saba is a Senior Research Scientist at the Institute for Experiential AI at Northeastern University.
Dr. Saba’s work focuses on advancing the understanding and modeling of language in AI, advocating for a bottom-up, symbolic approach to language processing .
Dr. Saba taught computer science at the University of Ottawa, the New Jersey Institute of Technology (NJIT), the University of Windsor, and the American University of Beirut (AUB) and has published around 50 technical papers including an award-winning paper presented at the German Artificial Intelligence Conference (KI-2008).
Dr. Saba has also worked with at AT&T Bell Labs, IBM, Cognos, and the American Institutes for Research as well as two startups where he was the Principal AI Scientist at Astound and the CTO of Klangoo, where he co-developed the Magnet digital content semantic engine.