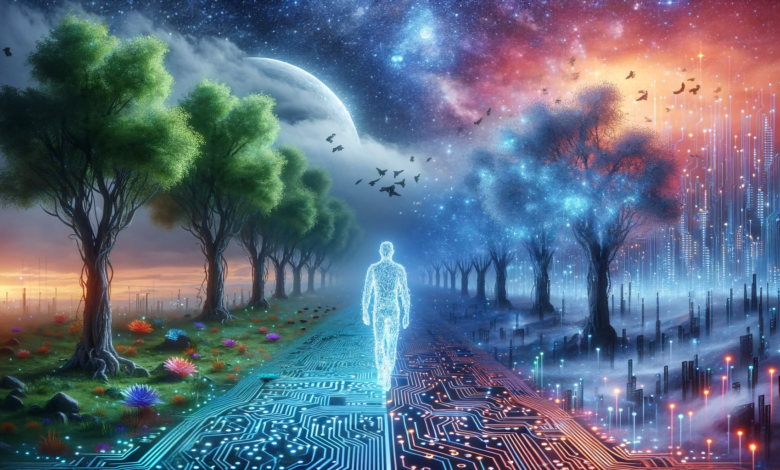
In today’s world of technology, artificial intelligence, or AI, is a real game-changer. It’s amazing to see how far it has come and the impact it’s making. AI is more than just a tool; it’s reshaping entire industries, changing our society, and influencing our daily lives in significant ways. However, with all groundbreaking technologies, AI comes with its own unique challenges. One such intriguing aspect is the phenomenon of AI hallucinations. This might sound a bit unusual, but it’s a crucial part of understanding the complexities of AI.
AI hallucinations occur when AI systems, like those used for generating images, writing texts, recognizing voices, or making decisions, produce results that are odd, unexpected, or don’t seem to align with the input they were given. These aren’t just minor glitches; they’re signs of deeper issues in the AI’s learning process and how it handles data. They reveal the limitations of current AI models and the hurdles in creating systems that can truly interpret the world in a human-like way.
This topic is important for everyone, not just those interested in tech. As AI becomes more integrated into various fields – healthcare, finance, creative industries, and even everyday consumer products – understanding AI hallucinations becomes increasingly vital. It’s about looking beyond just the technical details and considering the broader implications of AI in our lives. We need to explore how AI works, its reliability, ethical considerations, and its role in shaping our future. So let’s delve into this and unpack not just the mechanics of AI hallucinations but also their wider impact on our relationship with technology.
A Closer Look
- Unpredictable Outputs: AI hallucinations are characterized by their unpredictability. The AI may produce results that are drastically different from what a human would reasonably expect given the same input.
- Absence of Logical Consistency: These outputs often lack logical consistency, either with the real world or within the context of the provided data. For example, an AI might generate an image of a cat with three eyes, or a story where characters’ actions contradict earlier parts of the narrative.
- Disconnection from Input Data: Hallucinations in AI are notably disconnected from the input data. The AI might generate content that seems entirely unrelated to the input it was given, indicating a misunderstanding or misinterpretation of the data.
AI hallucinations are a window into the current limitations and challenges of artificial intelligence. Understanding these phenomena is crucial for developers and users alike, as it highlights the importance of robust training, ethical considerations, and realistic expectations of AI’s capabilities and limitations. As AI continues to advance, addressing the causes and implications of these hallucinations will be essential for developing more reliable and trustworthy AI systems.
The Underlying Causes of AI Hallucinations
Understanding the root causes of AI hallucinations is crucial for addressing the limitations of current AI technologies and guiding their future development. These causes are multifaceted, stemming from the inherent characteristics of AI systems, the data they are trained on, and the complexity of their learning algorithms.
Data Quality and Bias
- Inadequate Training Data: AI models learn from the data they are trained on. If this data is limited, unrepresentative, or biased, the AI is likely to develop skewed understandings and patterns. For instance, a model trained on a narrow range of images or text might not accurately interpret or generate diverse content.
- Data Noise and Errors: Training datasets often contain noise or errors. AI models might pick up and amplify these errors, leading to hallucinatory outputs. For example, a text generation model might learn from a dataset with typographical errors and start reproducing similar mistakes in its outputs.
The world according to Stable Diffusion is run by White male CEOs. Women are rarely doctors, lawyers or judges. Men with dark skin commit crimes, while women with dark skin flip burgers
HUMANS ARE BIASED. GENERATIVE AI IS EVEN WORSE, Bloomberg
Algorithmic Complexity and Overfitting
- Complex Neural Networks: Modern AI models, especially those using deep learning, involve highly complex neural networks. These networks can become excessively fine-tuned to the training data, a phenomenon known as overfitting. This overfitting can lead to the model performing well on training data but poorly on new, unseen data, resulting in hallucinatory or nonsensical outputs.
- Lack of Generalization: AI systems might struggle to generalize from their training to apply learned patterns in new contexts. This lack of generalization can result in outputs that are accurate within the context of the training data but bizarre or incorrect when dealing with new data or scenarios.
Absence of Contextual and World Knowledge
- Limited Understanding of Context: AI systems, particularly those based on machine learning, lack an intrinsic understanding of the real world and context. They process information based on identifying patterns in data, without a true comprehension of what those patterns mean. This limitation can lead to outputs that are contextually inappropriate or nonsensical.
- Inability to Discern Truth from Fiction: AI does not inherently understand truth or fiction. It treats all input data as equally valid for learning, which can result in the generation of outputs that are factually incorrect or fantastical.
Technical Limitations and Design Flaws
- Model Architecture and Design: The specific architecture and design of an AI model can predispose it to certain types of errors or hallucinations. For instance, certain text generation models might be more prone to losing coherence over longer text spans.
- Resource Constraints: Constraints in computational resources can also lead to AI hallucinations. For example, a model might be trained on a subset of available data due to resource limitations, leading to a less comprehensive understanding and, consequently, flawed outputs.
Interplay with Human Interaction
- Feedback Loops: AI systems often interact with human users, who can inadvertently reinforce hallucinatory patterns. For example, if users engage more with bizarre or sensational AI-generated content, the AI might learn to produce more of such content.
- Human Interpretation and Bias: The way humans interpret AI outputs can also play a role. Sometimes, what is perceived as a hallucination might be a result of human misinterpretation or expectation bias.
The underlying causes of AI hallucinations are diverse and interrelated, involving issues with training data, algorithmic design, and the fundamental limitations of current AI technology in understanding context and the real world. Addressing these challenges requires a multifaceted approach, including improving data quality, enhancing model design, and developing better methods for AI to understand and interpret context. As AI technology continues to evolve, understanding and mitigating these causes will be pivotal in advancing more reliable and effective AI systems.
Examples of AI Hallucinations
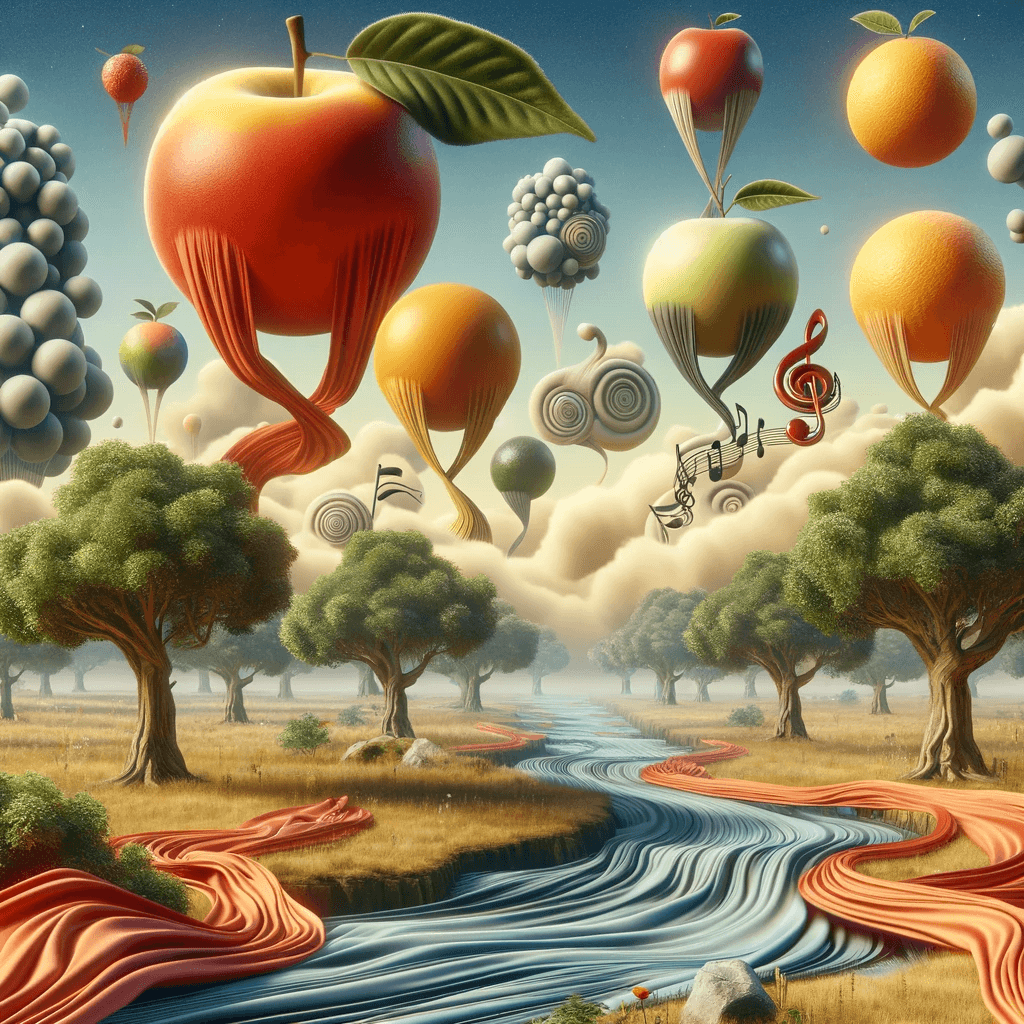
Exploring real-world examples of AI hallucinations provides a tangible understanding of this phenomenon. These instances demonstrate how AI, despite its advanced capabilities, can sometimes diverge from expected or logical outputs, offering a glimpse into the current limitations and quirks of these systems.
Image Generation Anomalies
AI models like DALL-E, designed to create images from textual descriptions, have produced some notably odd results. For example, when asked to generate an image of a “pineapple-shaped building,” the AI might create a structure that defies architectural norms, with surreal features that wouldn’t be feasible or functional in a real-world context. Such anomalies highlight the AI’s struggle to balance creativity with realistic interpretation.
In the picture above, the generated image above shows a surreal landscape with a sky filled with oversized fruits and trees with leaves shaped like musical instruments, alongside a silk-like river.
Text Generation Inconsistencies
AI systems like openAI GPT, Bard, Claude AI and others, which generate text based on input prompts, have shown a propensity for producing outputs that can veer off-topic or become nonsensical. For instance, an AI-generated article on a historical event might start accurately but then introduce elements or characters that never existed, reflecting the AI’s challenges in maintaining thematic and factual consistency.
Live Example
User Prompt:
“Can you tell me about the recent discovery of a new planet where life exists similar to Earth?”
AI Response (Hallucination Example):
“Certainly! Scientists have recently discovered a new planet, named Gliese 581g, located within the habitable zone of its star system. This planet has been confirmed to have conditions similar to Earth, including an atmosphere rich in oxygen and water bodies. The discovery marks a groundbreaking moment in astronomy and raises the possibility of extraterrestrial life, as this planet could potentially support human-like life forms.”
Analysis:
- Inaccuracy: As of my last update, there has been no confirmed discovery of an extraterrestrial planet with Earth-like conditions supporting life.
- Confidence in Incorrect Information: The AI presents the information as a fact, without any indication of uncertainty, which is misleading.
- Misleading Details: The mention of specific details like the name “Gliese 581g” and descriptions of the planet’s characteristics adds a layer of authenticity to the false information.
In this example, the AI has created a plausible but entirely fictional scenario. This illustrates the importance of cross-verifying AI-generated information, especially in fields like science and technology where accuracy is crucial.
Deepfake Videos and Voices
The creation of hyper-realistic deepfakes is another area where AI hallucinations manifest. There have been instances where AI-generated videos or voice clips are so convincing that they are indistinguishable from real footage or recordings. However, these deepfakes sometimes include subtle errors, like unnatural facial movements or voice modulations, which, while often imperceptible, reveal the AI’s inability to perfectly mimic human nuances.
Autonomous Vehicle Misinterpretations
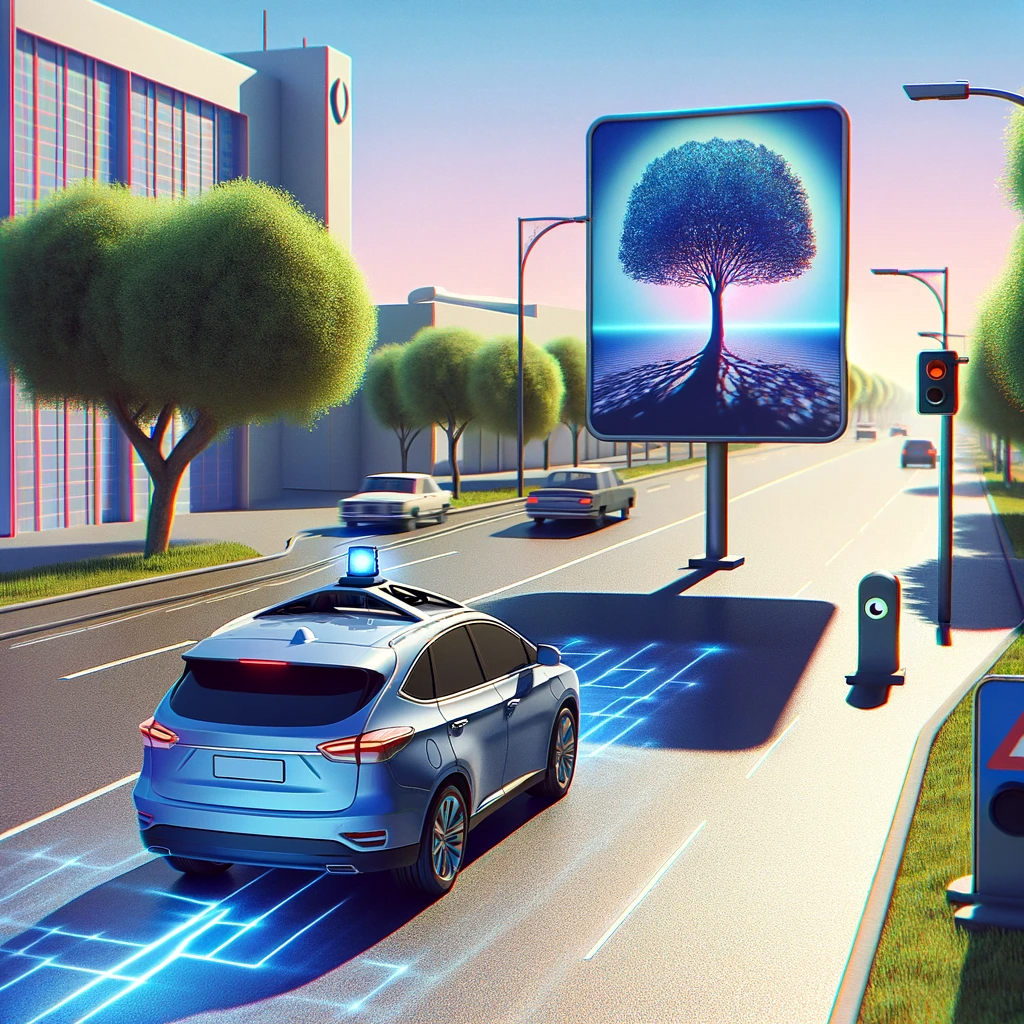
In the field of self-driving cars, AI systems have sometimes misinterpreted road signs or objects, leading to incorrect decisions. For example, an autonomous vehicle might misidentify a billboard as a real object on the road, or interpret shadows on the road as physical obstacles, showcasing the challenges AI faces in accurately interpreting complex visual environments.
AI in Healthcare Misdiagnoses
There have been instances in healthcare where AI systems, designed to assist in diagnosing diseases from medical imagery, have provided incorrect diagnoses. For example, an AI might misinterpret a benign growth as malignant, or vice versa, due to limitations in its training data or an inability to contextualize the image fully.
Voice Assistants’ Misunderstandings
Popular voice assistants like Google Assistant, Siri or Alexa have occasionally provided bizarre or unrelated responses to user queries. This can happen due to misinterpretation of the user’s speech or the AI drawing incorrect conclusions from its database, reflecting the challenges in natural language understanding and processing.
These examples across various domains illustrate the multifaceted nature of AI hallucinations. They highlight the importance of ongoing research and development to enhance AI’s understanding, reliability, and decision-making capabilities, ensuring these systems can be trusted and effectively integrated into our daily lives and critical industries.
Implications and Challenges of AI Hallucinations
AI hallucinations, while often intriguing, pose significant implications and challenges that extend across technological, ethical, and societal realms. Understanding these challenges is crucial for responsible AI development and deployment.
Ethical and Societal Implications
- Propagation of Bias and Stereotypes: AI hallucinations can unintentionally propagate biases and stereotypes. If an AI system is trained on biased data, it might generate outputs that reinforce these biases, leading to societal and ethical concerns.
- Misinformation and Trust: AI-generated content that is factually incorrect or misleading can contribute to the spread of misinformation. This is particularly concerning in the context of news generation, historical summaries, or educational content, where accuracy is paramount.
- Impact on Human Perception: AI-generated images, texts, or videos that are surreal or unrealistic might alter human perception or understanding of certain concepts or realities, especially if consumed without critical assessment.
Challenges in Critical Applications
- Reliability in High-Stakes Environments: In fields like healthcare, law, or finance, where decisions based on AI outputs can have significant consequences, AI hallucinations pose a major reliability issue. For instance, a misinterpreted medical image or a flawed financial forecast due to AI errors can lead to serious repercussions.
- Safety in Autonomous Systems: For autonomous systems like self-driving cars or automated flying drones, hallucinations in image or sensor data interpretation can lead to safety hazards, potentially causing accidents or failures.
Technological and Developmental Challenges
- Model Training and Validation: Developing AI models that are both powerful and reliable, capable of understanding context and minimizing hallucinations, is a significant technological challenge. This involves not only advancements in algorithms but also improvements in training methodologies and validation techniques.
- Data Quality and Management: Ensuring high-quality, diverse, and unbiased data for training AI systems is a major challenge, given the vast amount of data required and the potential for subtle biases or errors to creep in.
Legal and Regulatory Implications
- Accountability and Liability: Determining accountability for decisions made or actions taken based on AI hallucinations is complex. This raises legal questions about liability, especially when these decisions lead to harm or damage.
- Regulatory Compliance: Ensuring that AI systems comply with existing laws and regulations, particularly those related to privacy, data protection, and non-discrimination, becomes more challenging when dealing with AI hallucinations.
Public Perception and Trust in AI
- Erosion of Public Trust: Frequent or high-profile instances of AI hallucinations can erode public trust in AI technologies. This skepticism can hinder the adoption of beneficial AI applications across various sectors.
- Balancing Hype and Reality: Managing public expectations of AI capabilities, differentiating between what AI can reliably do and what remains challenging due to issues like hallucinations, is crucial for maintaining a balanced perspective on AI’s role in society.
AI hallucinations present a complex array of implications and challenges that need to be addressed through a combination of technological innovation, ethical consideration, educational outreach, and regulatory oversight. Understanding and mitigating these challenges is essential for harnessing the full potential of AI technologies while minimizing their risks and negative impacts. As AI continues to advance, ongoing dialogue and collaboration among technologists, ethicists, policymakers, and the public will be key to navigating these challenges effectively.
Artificial intelligence is not a substitute for human intelligence;
Fei-Fei Li
It is a tool to amplify human creativity and ingenuity.
Mitigating the Risks of AI Hallucinations
Addressing the risks associated with AI hallucinations requires a multi-pronged approach, involving technological advancements, ethical considerations, and regulatory frameworks. The goal is to enhance the reliability and trustworthiness of AI systems while minimizing their potential for unintended and potentially harmful outputs.
Enhancing AI Training and Development
- Diverse and Representative Data Sets: Ensuring that the data used to train AI models is diverse, comprehensive, and representative can help reduce biases and improve the model’s ability to generalize to new situations. This involves not just expanding the quantity of data but also carefully curating it for quality and variety.
- Advanced Model Testing and Validation: Rigorous testing and validation of AI models can help identify and mitigate hallucinations before deployment. This includes stress-testing models with unusual or edge-case scenarios to evaluate how they respond to unexpected inputs.
- Continuous Learning and Adaptation: Implementing continuous learning mechanisms where AI models can update and refine their understanding over time based on new data and feedback can help in reducing hallucinatory outputs.
Ethical Guidelines and Standards
- Developing Ethical AI Frameworks: Establishing clear ethical guidelines for AI development and use can help mitigate risks. These frameworks should address issues like fairness, transparency, accountability, and the potential impact of AI outputs on society.
- Bias Auditing and Mitigation: Regularly auditing AI models for biases and taking steps to mitigate any identified biases is crucial. This might involve incorporating ethical oversight during the development process and ongoing monitoring post-deployment.
Regulatory and Policy Measures
- Implementing Regulatory Frameworks: Governments and regulatory bodies can play a crucial role in mitigating the risks of AI hallucinations by establishing standards and regulations that guide the responsible development and use of AI.
- International Collaboration and Standards: Given the global nature of AI development, international collaboration is essential to establish and enforce standards that transcend national boundaries, ensuring consistent and effective mitigation strategies.
Public Awareness and Education
- Raising Awareness about AI Capabilities and Limitations: Educating the public, as well as AI developers and users, about the capabilities and limitations of AI, including the potential for hallucinations, is important. This can help set realistic expectations and encourage critical evaluation of AI-generated content.
- Promoting Digital Literacy: Enhancing digital literacy can empower individuals to better understand and interact with AI technologies, discerning between reliable AI outputs and potential hallucinations.
Collaborative Approaches
- Cross-Disciplinary Collaboration: Collaboration between technologists, ethicists, policymakers, and industry experts can lead to more comprehensive strategies for mitigating AI hallucinations, combining technical solutions with ethical and regulatory insights.
- User Feedback Mechanisms: Implementing mechanisms for users to provide feedback on AI outputs can help in identifying and correcting hallucinations. This feedback loop can be an invaluable resource for continuous improvement of AI models.
Technological Innovations
- Developing Explainable AI: Advancements in explainable AI (XAI) can help in understanding why an AI model might produce a hallucinatory output, making it easier to diagnose and fix underlying issues.
- Hybrid Models Incorporating Human Oversight: Combining AI with human oversight, especially in critical applications, can provide a safeguard against hallucinations, with humans able to intervene when AI outputs appear illogical or incorrect.
Mitigating the risks of AI hallucinations is a dynamic and ongoing process that requires a blend of technological innovation, ethical consideration, regulatory oversight, and public engagement. By addressing these challenges comprehensively, it’s possible to maximize the benefits of AI while minimizing its risks, paving the way for more trustworthy and reliable AI systems.
Mitigating AI hallucinations is akin to navigating a complex labyrinth; it demands a harmonious blend of robust data integrity, meticulous algorithmic oversight, and continuous, enlightened human engagement. In this intricate dance of technology and human insight, lies the key to unlocking AI’s true potential while anchoring it in the realm of reality
Conclusion: Navigating the Future of AI with Awareness and Responsibility
As we stand on the brink of a new era in artificial intelligence, the phenomenon of AI hallucinations serves as a poignant reminder of both the incredible potential and the inherent limitations of these technologies. These anomalies, while often seen as mere curiosities or technical glitches, actually hold profound significance for the trajectory of AI development and its integration into the fabric of society.
Reflecting on the Journey and Challenges
The journey of AI, from its conceptual beginnings to the advanced systems we see today, has been marked by remarkable achievements and unexpected challenges. AI hallucinations, in particular, highlight critical areas requiring attention: the quality of training data, the complexity of algorithms, the need for contextual understanding, and the ethical ramifications of AI outputs.
The Imperative of Responsible Development
The mitigation of AI hallucinations is not just a technical challenge; it’s a responsibility that falls on the shoulders of developers, ethicists, policymakers, and users alike. It calls for a balanced approach that embraces innovation while conscientiously addressing potential risks. This includes enhancing AI training methods, establishing ethical guidelines, implementing regulatory frameworks, and fostering public awareness and education.
Embracing AI with Informed Optimism
As we move forward, it is essential to embrace AI with an informed optimism. Understanding the capabilities and limitations of AI, including the propensity for hallucinations, allows for a more realistic and grounded perspective. This awareness guides better decision-making, both in the development of AI technologies and in their application across various sectors.
Collaboration and Dialogue: Key to Progress
The future of AI will be shaped not just by technological advancements but also through ongoing dialogue and collaboration. By bringing together diverse perspectives from technology, ethics, law, and society, we can navigate the complexities of AI, including issues like hallucinations, in a way that maximizes benefits while minimizing harm.
Looking Ahead: A Future Augmented by AI
The potential of AI to augment human capabilities and transform industries is immense. As we continue to explore this potential, addressing phenomena like AI hallucinations will be crucial in ensuring AI systems are reliable, trustworthy, and beneficial. With a commitment to responsible development and a proactive approach to challenges, we can harness the power of AI to create a future that is not only technologically advanced but also ethically sound and socially responsible.
Final Thought
In conclusion, AI hallucinations, while representing a challenge, also offer an opportunity,an opportunity to refine our technologies, to sharpen our ethical frameworks, and to deepen our understanding of artificial intelligence. As we navigate this landscape, our focus should remain on developing AI that is not only powerful and innovative but also responsible and beneficial for all.