Welcome to LlamaCon 2025: Powering the Future of Open Source AI
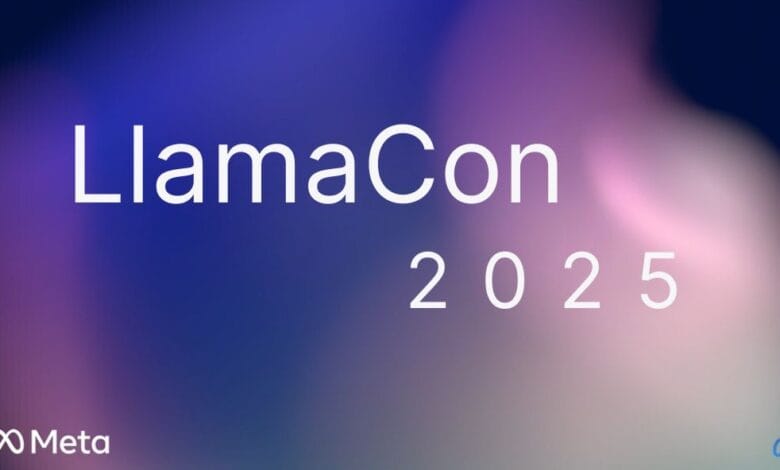
LlamaCon is Meta’s first-ever developer conference dedicated specifically to its open-source generative AI initiatives, particularly the Llama family of models. It signifies a dedicated focus on AI, separating these discussions from Meta’s broader Connect conference, which focuses more on on XR and metaverse technologies. The company’s annual Meta Connect conference, is scheduled separately for September 17-18, 2025.
LlamaCon 2025:
- Date: The inaugural LlamaCon took place today, April 29th, 2025.
- Location: The event’s held in person at Meta HQ in Menlo Park, California, with select sessions live-streamed online via the Meta for Developers Facebook page.
- Focus: The conference centered on the growth and momentum of Meta’s open-source Llama collection of models and tools. It aimed to share the latest developments in open-source AI to help developers build applications and products. The event celebrated the open-source community and showcased the Llama model ecosystem.
- Key Sessions & Speakers:
- A keynote address featured Meta’s Chief Product Officer Chris Cox, VP of AI Manohar Paluri, and research scientist Angela Fan. They discussed developments in the open-source AI community, updates on Llama models and tools, and previews of upcoming AI features.
- Conversations included Meta CEO Mark Zuckerberg speaking with Databricks CEO Ali Ghodsi about building AI-powered applications.
- Mark Zuckerberg also will have a discussion with Microsoft CEO Satya Nadella about AI trends, real-world applications, the future of open-source AI, and developer ecosystems.
- Announcements: Meta announced the Llama API, available as a limited free preview, providing developers a platform to experiment with Meta’s AI models, including Llama 4 Scout. While Meta had recently released the Llama 4 family of models (Scout and Maverick) prior to the conference, the event provided a platform to discuss these advancements and future plans.
The LlamaCon 2025 opening session kicked off with a welcome from Meta Chief Product Officer, Chris Cox. Reflecting on the past two years, Cox noted how open source AI was initially viewed skeptically, with concerns about financial viability, potential distractions, and safety implications, such as falling into the hands of “bad guys”. There was also doubt about whether open source could compete with closed labs in terms of performance.
However, Meta, drawing on its history of building on and contributing to open source stacks (including web, mobile, and AI toolkits), believed in its potential. Cox stated that there is now general consensus in government, the tech industry, and among major labs that open source is here to stay. He highlighted that open source AI can be safer and more secure because it can be audited and improved in broad daylight, similar to Linux. Furthermore, it can perform at the frontier and, crucially, be deployed and customized for specific use cases and developers.
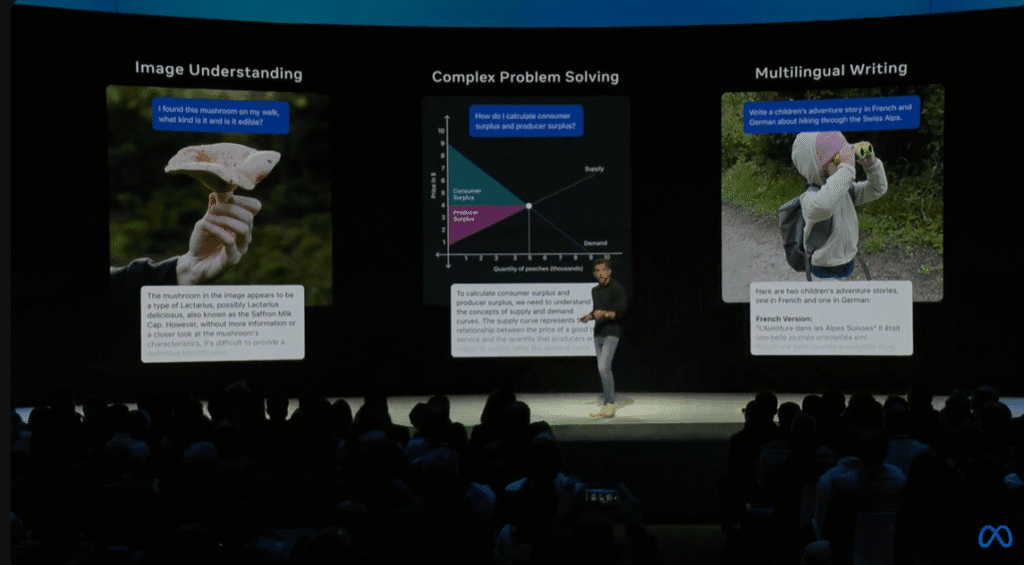
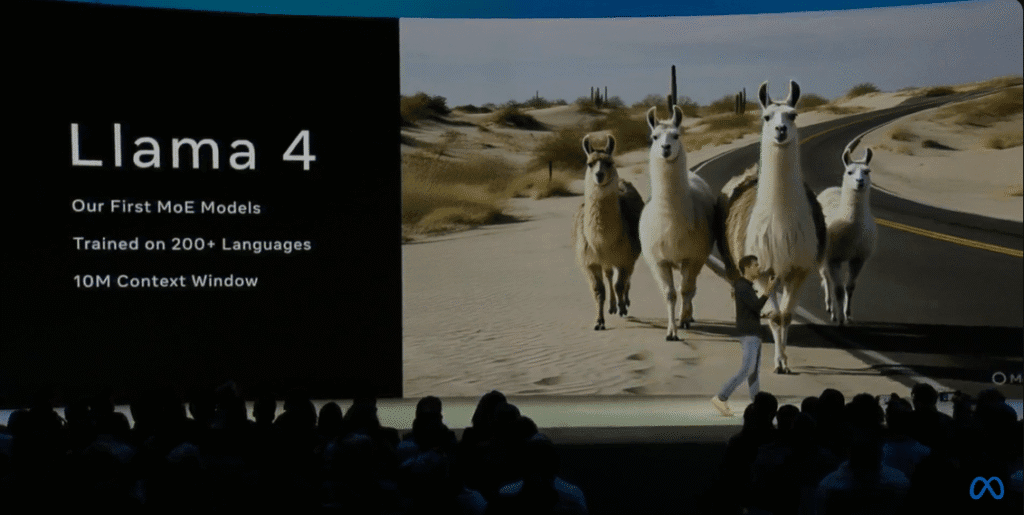
Llama’s Explosive Growth and Developer Ecosystem
The session highlighted the tremendous growth and support for Llama. Just ten weeks before LlamaCon 2025, Llama 4 had reached 1 billion downloads. Excitingly, that number had grown to 1.2 billion downloads in just ten weeks. This growth is significantly driven by the community, with most downloads on platforms like Hugging Face being Llama derivatives. There are thousands of developers contributing tens of thousands of derivative models, supporting various languages and use cases.
This success is also attributed to awesome partners who have made it easier for developers to get started with Llama. The Llama stack is designed to be modular and easy to plug and play into applications, allowing developers to choose the best parts of solutions and switch components easily, simplifying development.
Innovations in Llama 4 Models
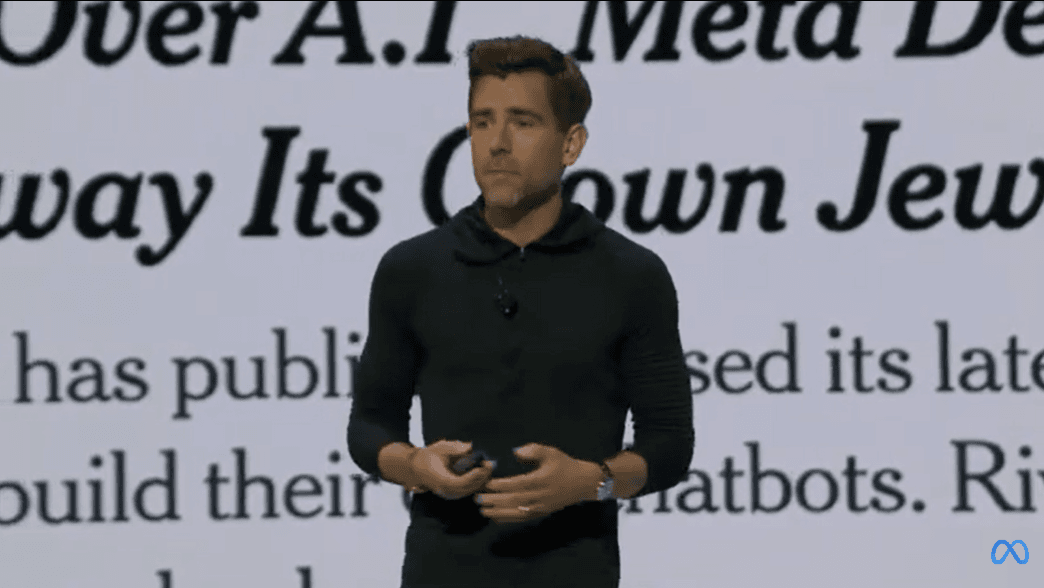
Meta was very focused on community feedback when developing Llama 4. Key areas of focus included:
- Performance in a small package: Llama 4 is the first Mixture of Experts (MoE) model from Meta, designed to offer more performance in a smaller binary. This architecture allows for faster and cheaper inference with more intelligence.
- Multilingual Support: Llama 4 is massively multilingual, natively supporting 200 languages and using 10x more multilingual tokens than Llama 3.
- Huge Context Window: The context window is large enough to include entire codebases or large documents like the U.S. tax code.
- Visual Reasoning: Significant improvements were made in visual reasoning for tasks like OCR, processing PDFs, charts, and graphs, addressing a major request from developers. This commitment to visual domains is tied to the belief that AI, like people, will learn by seeing and interacting with the world.
- Model Sizes and Efficiency: The goal was to get as much intelligence as possible into the smallest models for scalability and business use.
- Llama 4 Scout is a smaller model designed to run on a single H100 GPU, excelling on benchmarks for its size.
- Llama 4 Maverick is the next size up (17 billion active parameters), used in Meta’s production environments, including powering Meta AI. Its performance was boosted using a massive teacher model called Llama Behemoth.
- Price per Performance: Meta is proud of Llama 4’s price per performance, which measures cost per token, latency, and intelligence (using composite benchmarks). This focus is critical for deploying models at scale, whether for small or large businesses.
The Llama API: Speed, Ease, Customization, No Lock-in
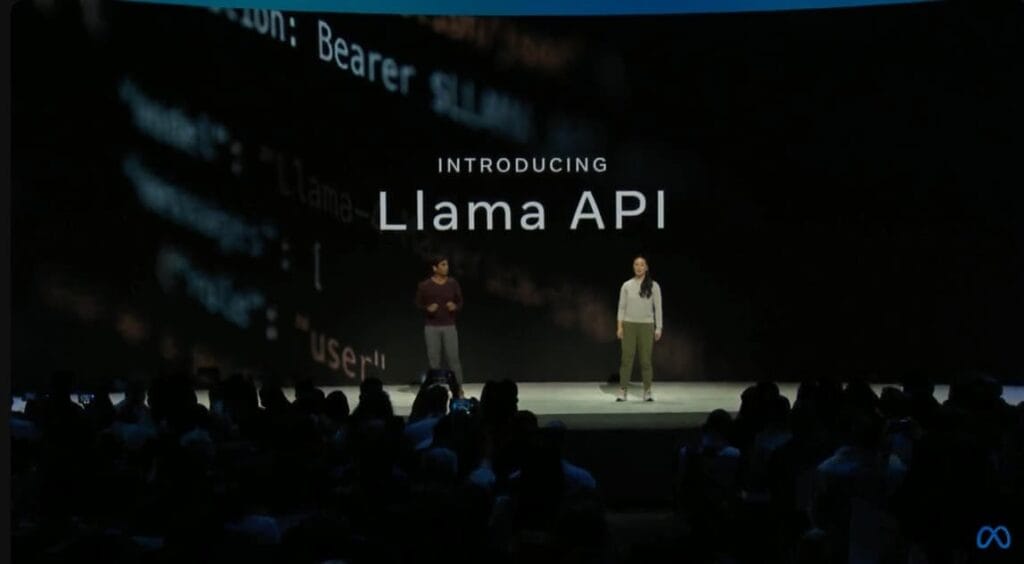
A major announcement was the release of the Llama API, allowing developers to start using Llama with just one line of code. The goal of the API is to be the fastest and easiest way to build with Llama, while also providing the best way to customize models without lock-in.
Key features and benefits of the Llama API include:
- Ease of Use: Registering with one click, making curl requests, and compatibility with the OpenAI SDK by adjusting the endpoint simplifies migration.
- Chat Completion Playground: A playground is available for quick experimentation with different models, settings (like system instructions and temperature), and configurations.
- Multimodal Capabilities: The API supports image input, including sending multiple images per prompt, leveraging Llama 4’s leading multimodal LLM capabilities.
- Structured Responses & Tool Calling: Developers can input JSON schemas for structured responses and specify tools (a preview feature seeking community feedback).
- Data Privacy and Security: Input sent to the API and generated output are not used to train Meta’s models.
- Fine-tuning and Customization: The API is designed for fine-tuning models for specific product use cases. Developers have full agency and control over these custom models and can download and run them anywhere, ensuring no lock-in. Synthetic data toolkits are available to help generate post-training data.
- Evaluation Tools: An evaluation area allows developers to assess the performance of their fine-tuned models, using data splits and configurable graders to score model output against specific criteria (like factuality). The evaluation flow aims to be simple compared to alternatives.
Early feedback on the Llama API from start-ups testing it highlights its ease of use, speed, and quality of responses.
Efficiency and Inference Stack
Efficiently serving models at scale is crucial, especially since Llama 4 Maverick powers Meta AI. The Mixture of Experts (MoE) architecture was chosen with efficiency in mind, activating only a fraction of the total parameters. Maverick’s size was specifically chosen to make deployment straightforward, allowing for single-host inference while distributed options are available for higher throughput. The smaller Scout model is designed to run on a single H100.
The inference stack utilizes a new MOE runtime. New features shipped with it include:
- Speculative Decoding: Uses a smaller model to draft tokens, speeding up token generation by about 1.5x to 2.5x.
- Paged KV Cache: Handles long queries efficiently by breaking the KV cache into smaller blocks for better memory assessment.
Deep integration with hardware makers is possible due to the open model nature, allowing for better performance. Collaborations with Cerebras and Groq were announced, allowing developers to request experimental access to Llama 4 models powered by their hardware for faster speeds and streamlined prototyping.
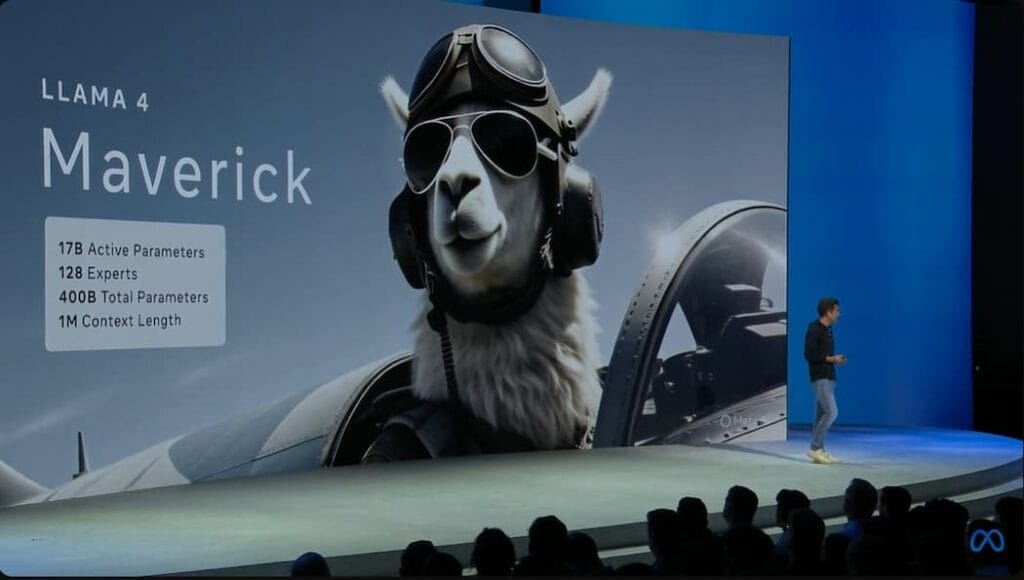
Use Cases, Meta AI, and the Move to Visual Domains
The session showcased diverse use cases demonstrating the possibilities with Llama:
- Local Deployments: Llama is being used in space by Booze Allen and the International Space Station, providing astronauts and scientists with local access to documentation without a connection to Earth.
- Privacy-Sensitive Domains: In medicine and health care, Llama is helping doctors reduce paperwork and understand complex cases by analyzing large amounts of literature.
- Regional Applications: Farmer Chat in Sub-Saharan Africa provides real-time agricultural information to farmers. Kovac, a used car marketplace in Latin America and the Middle East, uses Llama for customer support based on good fine-tunes for specific regions and content.
- Enterprise: AT&T is using Llama to analyze support call transcripts to identify bugs for engineers, achieving good results by customizing the model to their specific needs. Box, serving 100,000 customers, partnered with IBM to scale enterprise use cases, highlighting the benefits of open source for data security.
- Consumer: Llama 4 powers Meta AI, used by almost a billion monthly users across apps like WhatsApp and standalone experiences like on Ray-Ban glasses. Meta announced a standalone Meta AI app focused on pushing the limits of AI usage, particularly the voice experience. Features include low-latency, highly personalized voice (remembering details like kid’s names and birthdays by connecting accounts). An experimental “duplex voice” mode allows for native audio output and dynamic dialogue like a phone call. The app also emphasizes sharing prompts and AI creations to inspire users. Meta AI is paired with Ray-Ban glasses, which are described as the “ultimate AI device” due to their multimodal and voice interface capabilities.
Open Source, Distillation, and the Future of AI
Mark Zuckerberg joined the session to discuss the landscape of open source AI with Ali Ghodsi, CEO of Databricks. Ghodsi emphasized the rapid progress in AI, noting how smaller Llama models quickly surpassed larger models from just a year prior. He highlighted the impact of MoE architectures for speed and cost reduction, and the increase in context length for new use cases.
Both leaders agreed on the transformative impact of open source AI. Key points included:
- Pricing Pressure: Open source models, like Llama, put significant pressure on the price of serving large models. This lower price unlocks many new use cases for organizations. Zuckerberg noted that other companies drop API prices every time Meta releases a new Llama model.
- Accelerated Research and Progress: Open source allows universities, researchers, and the whole world to work on AI models, leading to much faster progress compared to closed source systems where progress depends on a single company.
- Community and Ecosystem: Open source fosters a community that builds an ecosystem around the models, allowing for mixing, matching, and experimenting with different approaches. Ghodsi mentioned seeing developers on platforms like Reddit doing “crazy things” with Llama derivatives.
- Distillation and Customization: A surprising trend observed is the focus on distillation – taking larger, smarter models and compressing their intelligence into the smallest possible model needed for a specific, repetitive task. This is driven by the need for cost efficiency and low latency for specific applications. Distillation is seen as an invaluable way to get the intelligence needed in the desired form factor. Databricks has developed techniques like TOA (Reinforcement Learning on Customer Data) to help models understand and reason on specific enterprise data. Such reinforcement learning techniques would not be possible with closed source models.
- Mixing and Matching: With multiple open source models available (like DeepSeek, Qwen, alongside Llama), developers can mix and match the best capabilities from different models to create exactly what they need. Ghodsi noted the popularity of “Llama distilled DeepSeek ones” where reasoning from DeepSeek was distilled onto Llama models. This mix-and-match capability makes open source an “unstoppable force” but requires infrastructure like Databricks provides.
- Reasoning Traces: Zuckerberg discussed the interesting idea of making reasoning traces open source, as the data used to train reasoning models (like math or coding problems with verifiable answers) is less proprietary than training data derived from services. This could be a new type of open source contribution. Ghodsi noted research showing distillation of reasoning traces can be very inexpensive.
- Safety in Customization: Both leaders acknowledged the importance of safety and security when customizing models, especially when mixing models from different origins. Tools like Llama Guard and Code Shield (both open-sourced by Meta) are part of addressing this.
- The Future of Agents: There is immense excitement around AI agents, particularly for businesses (like customer support and sales agents for small businesses using Meta’s services). The need for customized agents that won’t, for example, recommend a competitor’s product highlights the need for many different AIs rather than just one. Currently, quality and accuracy are the main focus for agents before widespread deployment. Human supervision is still seen as necessary for many agents.
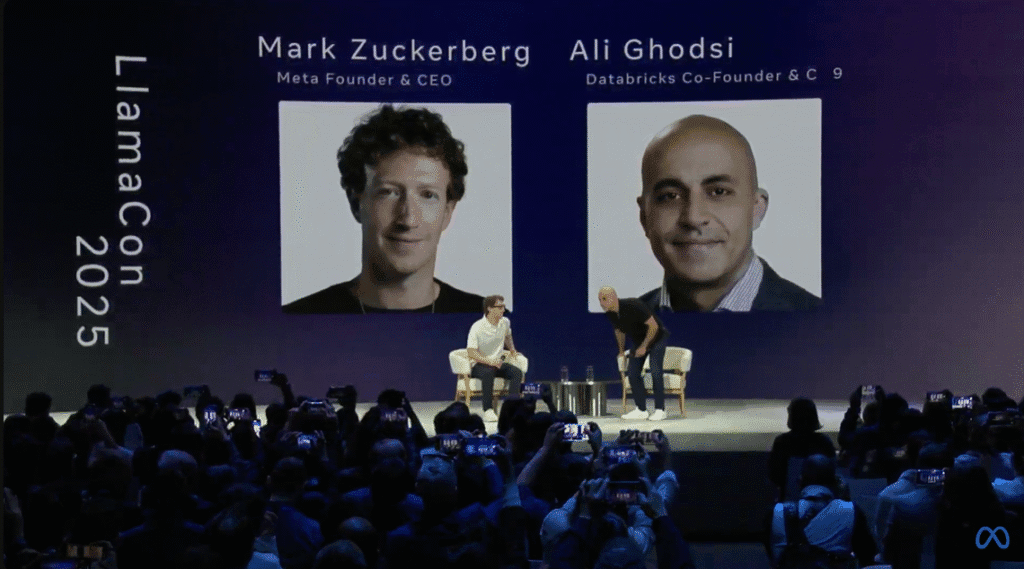
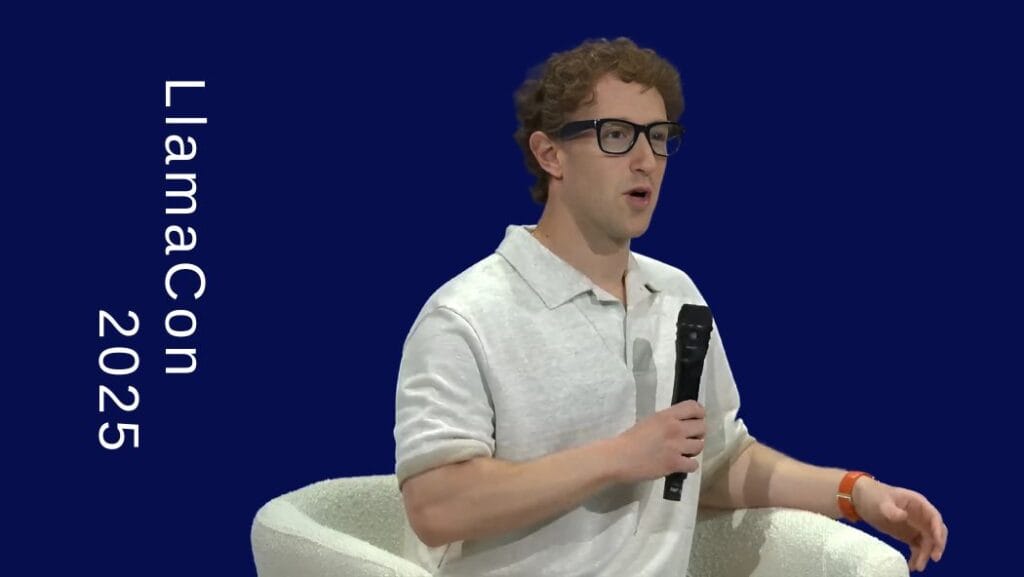
Advice for Developers
Ali Ghodsi offered advice for developers, emphasizing that it’s still “Day 0 of the AI era” and the most amazing applications are yet to be invented. He encouraged exploration and use of the models. He stressed that having a data advantage and being able to tune or distill open source models on that specific data set is key to building use cases where the model excels. Finding a data flywheel where usage improves the model on the specific task can lead to significant innovation. Ghodsi also highlighted the importance of building evaluations (evals) and benchmarks for the specific use cases being developed, as you can’t manually check every prediction. He concluded by encouraging developers to try out new ideas and customize models to become really good at whatever use cases they have in mind.
The session ended with thanks for the community’s collaboration and anticipation for what developers will build with Llama 4 and the API.ore crazy ideas, and focus on data, custom evaluations, and customization.